If you are thinking about replacing your language team with AI, you might want to hold off that decision for a while. Although AI technology has grown by leaps and bounds in previous years, so much so that it has overtaken human ability in areas like disease diagnostics and driving cars, it still falls short when it comes to language. This isn’t to say that language AI isn’t powerful- quite the contrary. Machines can translate large documents instantaneously, carry on simple conversation, produce written texts and do a myriad of other things that make our day-to-day lives easier. But despite this, experts say that full reliance on AI for language is still a far way off. Humans are the bedrock of language and will remain so into the foreseeable future, meaning that your decision to go full AI might be a bit premature.
So why are humans so instrumental in language-based AI? And what have machines yet to conquer? In this post, we discuss the indispensable contributions that humans make to language and why their inclusion in your language projects is vital for clear and high-quality output.
1. Humans understand context
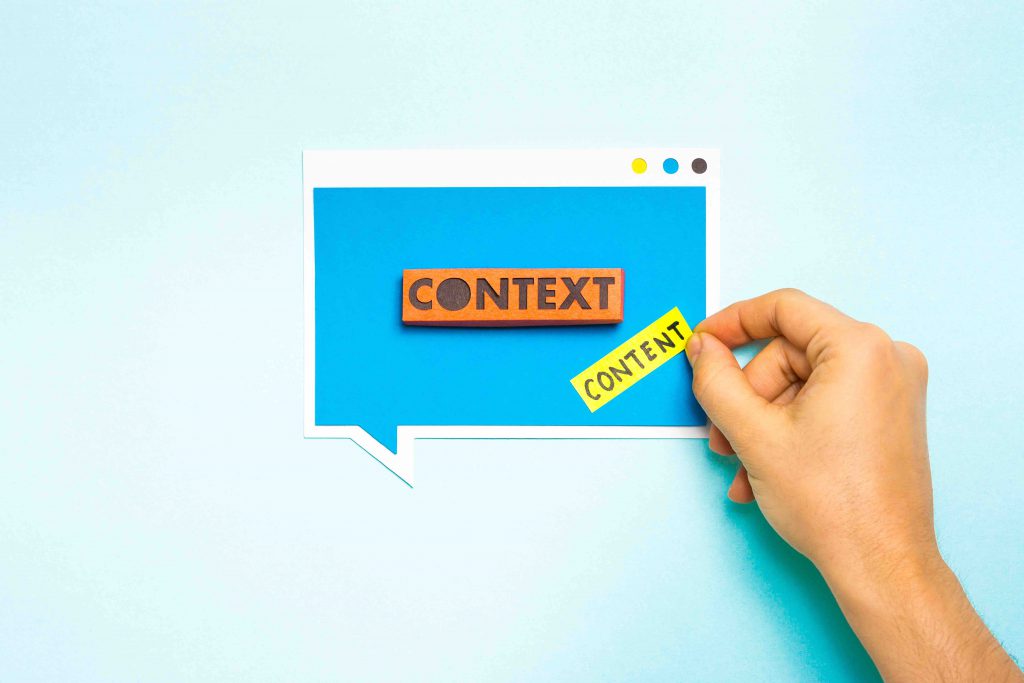
Language is extremely subjective, which means that its usage can vary from place to place, even down to an individual level. How words are used can greatly alter their original meaning, giving them new depth and intent. Humans are great at picking up these subtleties because we can read non-verbal cues, tones, and other nuances to understand what the text aimed to convey. AI software on the other hand is greatly rooted in objective reality that makes it follow a rigid set of rules (physical or mathematical) that governs its decision making.
This dependency on highly sophisticated systems trained on a vast amount of data makes AI very competent in some specific tasks. But because language is difficult to boil down to a rigid set of rules, developers may find it hard to design consistently accurate programs. It is wrong to say that languages don’t have rules- think grammar and conjugation- but these rules are driven by convention, not objective reality. Given that language also evolves, training AI can be likened to trying to score a goal on a shifting goal post. Additionally, only humans can decipher whether a text sounds natural or not. Machines may generate semantically accurate text, but it may be too robotic-sounding rendering the text ineffective.
Why is context important in language?
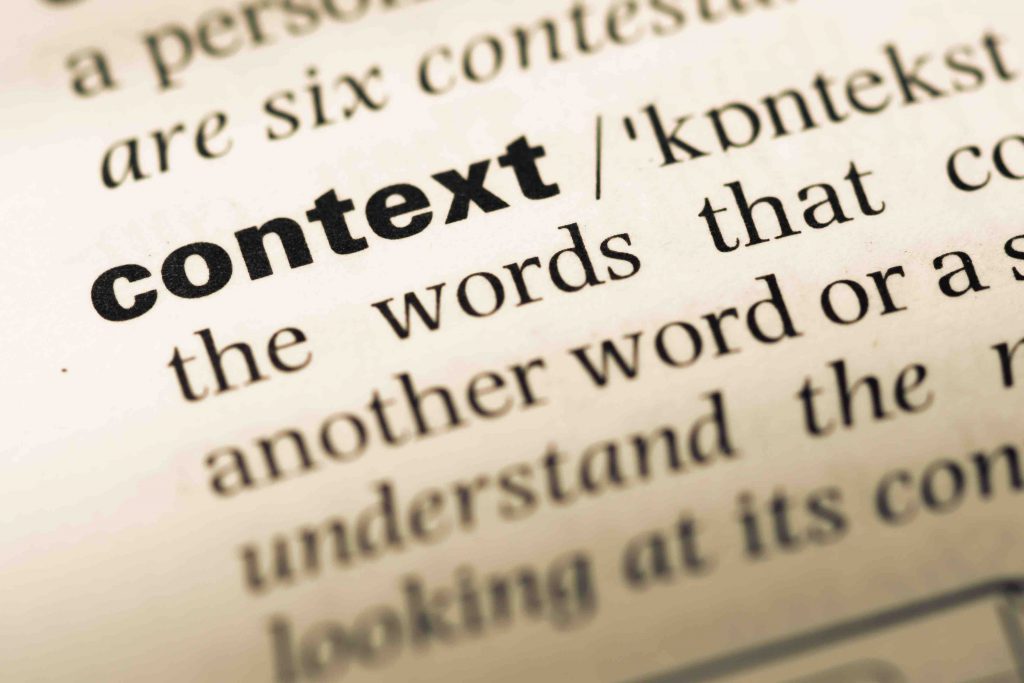
Up until recently, computers were unable to generate cohesive texts but thanks to natural language processing (NLP), driven by deep learning and statistical patterning, it is now the order of the day. This is why language tech has found mainstream success helping to build chatbots and voice assistants that you use in your day-to-day and that you may also employ in your business. But recent studies have shown that machines may not really understand what they read or write, which is what makes them more fallible. These pitfalls can make it hard for you to effectively rely on chatbots to communicate with your clients since they can make some glaring mistakes that not only exasperate your customers but also cause you to lose business especially if you don’t have the personnel to pick up the slack.
To demonstrate this, some researchers created a test to evaluate the reasoning of NPL systems using 44,000 questions grouped into identical pairs of sentences, except with one word flipped (trigger word) that gives the sentences different meanings. For example:
● The town councilors refused to give the demonstrators a permit because they feared violence.
● The town councilors refused to give the demonstrators a permit because they advocated violence.
The two sentences have varied meanings, and the machines had to decide who the subject in each case was. In the first case, it was the councilors and in the second the demonstrators. The results showed that even state-of-the-art models could not pass the test, with scores between 59.4% and 79.1%, contrasted with human subjects who scored an average of 94%. These results show that machines are not yet conversant with context as it applies to language.
The importance of context in translation
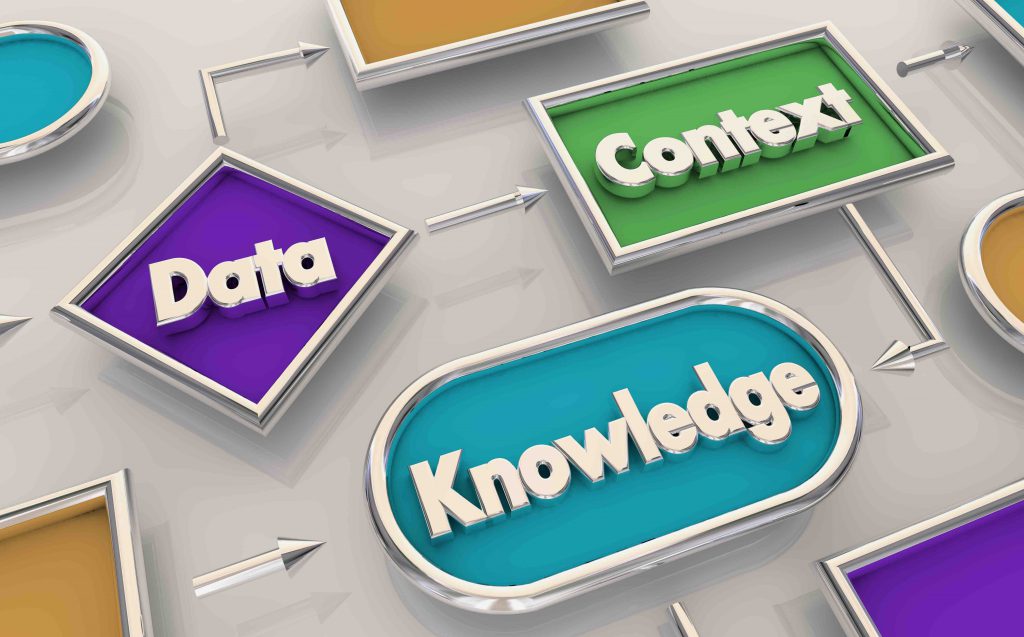
Translation is even shakier ground for language AI. Although some situations can make the use of machines appropriate, like the translation of lengthy manuals where there’s scant room for errors, it still falls short in other scenarios. If your original texts contain slang or local dialects, machines may be unable to translate it accurately. Also, where some cultural norms need to be adhered to in translation, machines find themselves out of depth. For example, in Germany and Japan, only formal language should be used in business scenarios, a nuance that machines may be unable to pick up on. To overcome this hurdle, most LSPs employ machines for the first phase of translation then finally use language experts to correct any errors, in a system referred to as Machine Translation Post Editing (MTPE). This way translation is faster with minimal errors.
2. AI is biased
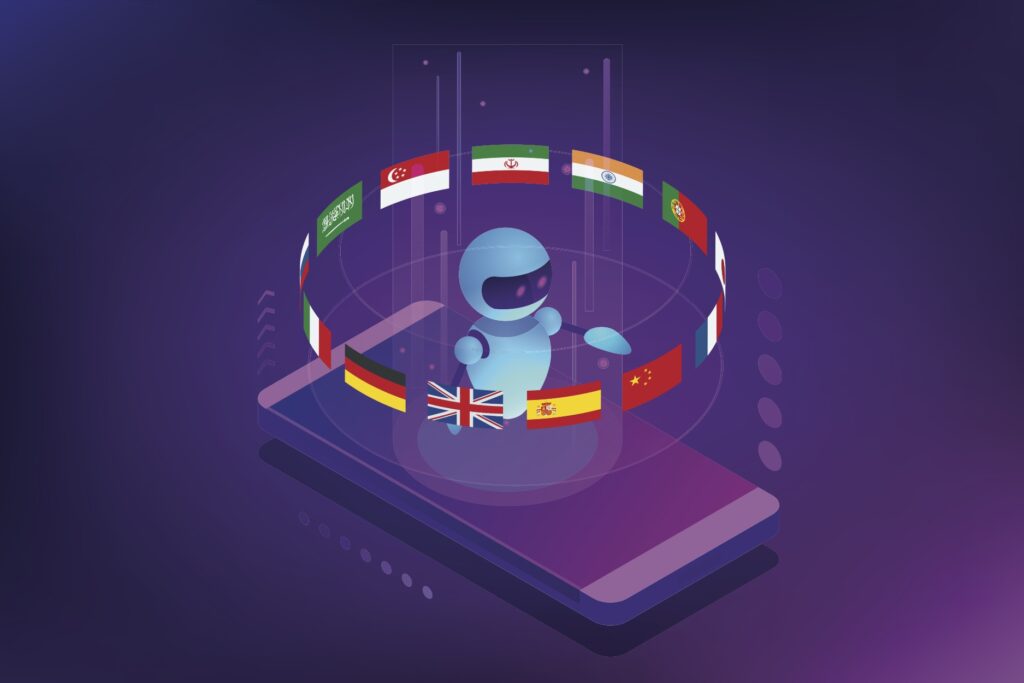
Although natural language processing has come into its own in the recent past, it has revealed that language algorithms teeter towards biased responses. A study conducted on a language model (GPT-3) showed that it returned responses that showed gender bias.
The program was fed with leading sentences like:
● He was very….
● He would be described as…..
Then the predictive texts generated were analyzed to show the common adjectives and adverbs chosen for the genders. The results showed that the program chose words that described the female’s appearance (“petite”, “gorgeous”) while for men it returned more general terms like “large” or “personable”.
The presence of bias in AI is nothing new, not just in gender but in race as well. Recently, face recognition software creators came under fire for creating algorithms that failed to register non-white faces. In these two cases, the machines are not to be blamed. The outputs generated as a result of the data being fed into the system that reflects the prejudices that are present in human interactions.
Mitigating biases in language-based AI
Research has shown that machines cannot detect biases as well as show why or how they are harmful. This is why humans need to be kept in the machine learning loop if there is any hope to reduce prejudice in language software.
Since technology like GPT-3 and other language bots are used in real-life scenarios, it can have extensive impacts on the well-being of people in society. The analysis of bias in AI is not only geared towards perfecting the end product, it is also about mitigating the damage that real people may suffer at the hand of biased technology. As language is a human construct, only humans have the power to affect the change that can propel the society towards a more tolerant, equitable, and accepting future. Also, because AI systems have shown their propensity towards fake news propagation and toxic language generation, which can influence actual events, human beings have to be involved to ensure that they are used responsibly.
3. Machines lack a sense of humor
Adopting neural networks in the place of phrase-based statistical systems was one of the major turning points for machine-based translation. It has led to a general improvement in quality and speed of translation. However, neural machine translation requires larger data sets than its predecessors to function properly. But the only large bilingual texts available are from official documents and religious texts that use dry, straightforward, procedural language that lacks wordplay, nuanced cultural references, and puns. Language algorithms based on these texts are as a result difficult to use in situations where highly colloquial language is used.
Picking up on jokes and innuendos is challenging even for human translators but they can rely on other crutches like body language or intent to get the intended meaning. This same feat is currently impossible for machines. When faced with text it doesn’t recognize for example, Google Translate offered unrelated translations, an error that experts pegged to the machine’s preference for fluency over accuracy.
The substitution of texts by machines can have far-reaching consequences in the case where the discourse of the translation is blamed on the initial text instead of a glitch in the translation software.
Humans bridge the gap in language algorithms
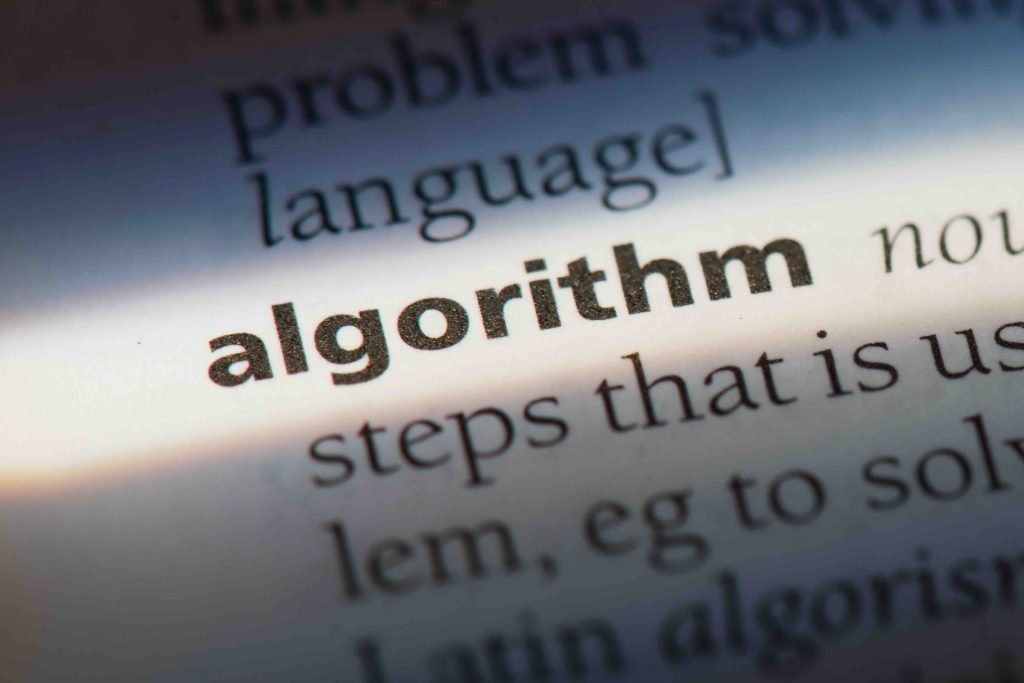
The greatest shortcoming of language models is that they are trained exclusively on texts that have no grounding in the real world. What they know is solely based on the texts they are trained on. You can have a simple back and forth with AI assistants like Alexa and Siri because your interactions are based on a narrow set of conditions, with limited vocabulary and in a controlled environment. But put to a task like that of interpreting live speech adds new layers of complexity that most programs may fail at overcoming.
Although there is much debate about whether machines can deduce meaning from pure text – most experts are leaning on the side that they don’t – one thing is clear: the involvement of human beings in language cannot be second-guessed. For ethical responsibility, as well as the integrity of outputs, AI language models still require heavy human supervision.
Ready to get the most out of artificial and human intelligence? Find out more about Tarjama language solutions that are powered by human creativity and AI speed.
Check out a sample of our professional translation solutions in action with our Arabic version of this blog.
Read Arabic BlogReferences
https://www.weforum.org/agenda/2018/10/3-reasons-why-ai-wont-replace-human-translators-yet/
https://www.technologyreview.com/2020/01/31/304844/ai-common-sense-reads-human-language-ai2/amp/
https://insidebigdata.com/2020/11/11/why-humans-still-need-to-be-involved-in-language-based-ai/