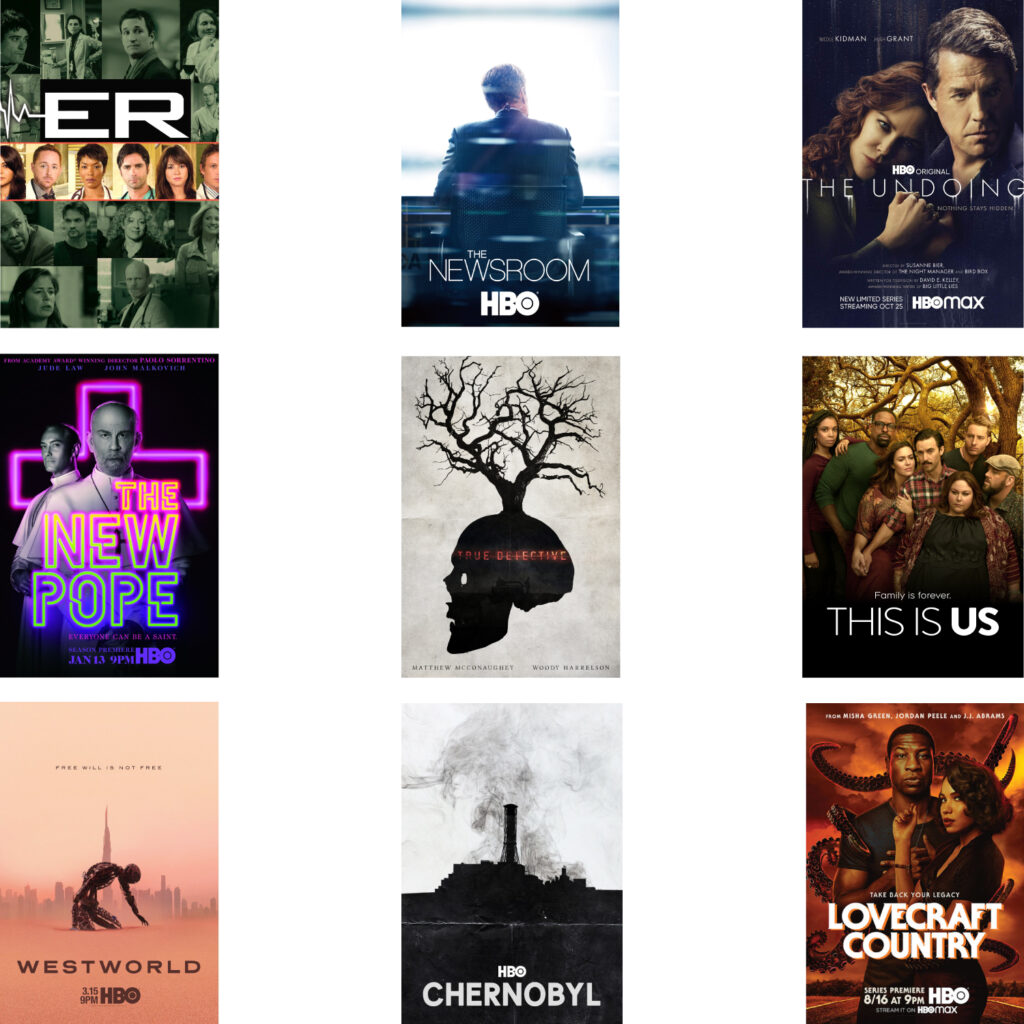
A top priority for OSN is to provide seamless entertainment for Arabic users on its platform. However, with the entertainment industry comes a unique set of challenges:
– Fast-paced environment
– Aggressive deadlines
– Security risks for exclusive content
– Too much content; too little time
– No room for error
Since 2009, Tarjama has been successfully partnering with OSN, providing them with localized subtitles for top-hit shows across 15+ genres.
Find out the unique challenges of some of the major shows – including Game of Thrones, Saturday Night Live, and Grey’s Anatomy – and how Tarjama helped conquer them.